Reducing False Positives in eCommerce Fraud Detection
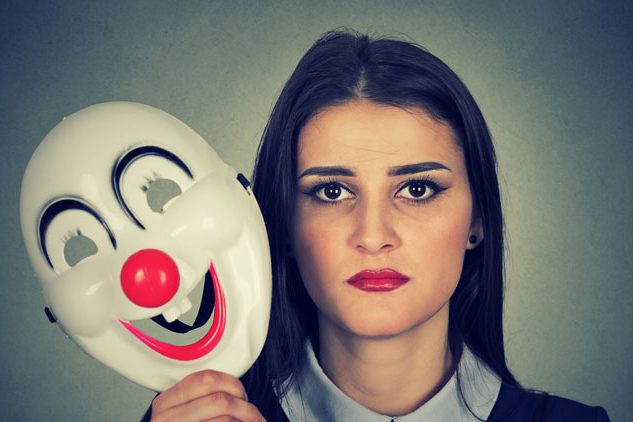
Fraud detection stands as a critical frontier in safeguarding businesses and individuals against financial malfeasance. As technology advances, so do the methods employed by fraudsters, necessitating robust fraud prevention systems to identify and thwart illicit activities. Businesses must avoid the various types of eCommerce fraud, ranging from chargebacks to account takeover fraud, especially in card-not-present situations. However, within the realm of credit card fraud detection, the phenomenon of false positives looms as a formidable challenge.
These instances, where legitimate transactions are mistakenly flagged as fraudulent, have significant repercussions for businesses and individuals alike. This article delves into the intricate world of false positives in fraud detection, aiming to unravel their complexities, understand their implications, and explore strategies for mitigation. By shedding light on this crucial aspect of fraud prevention, we aim to enhance awareness and empower stakeholders to strike a balance between precision and recall in their efforts to combat fraud.
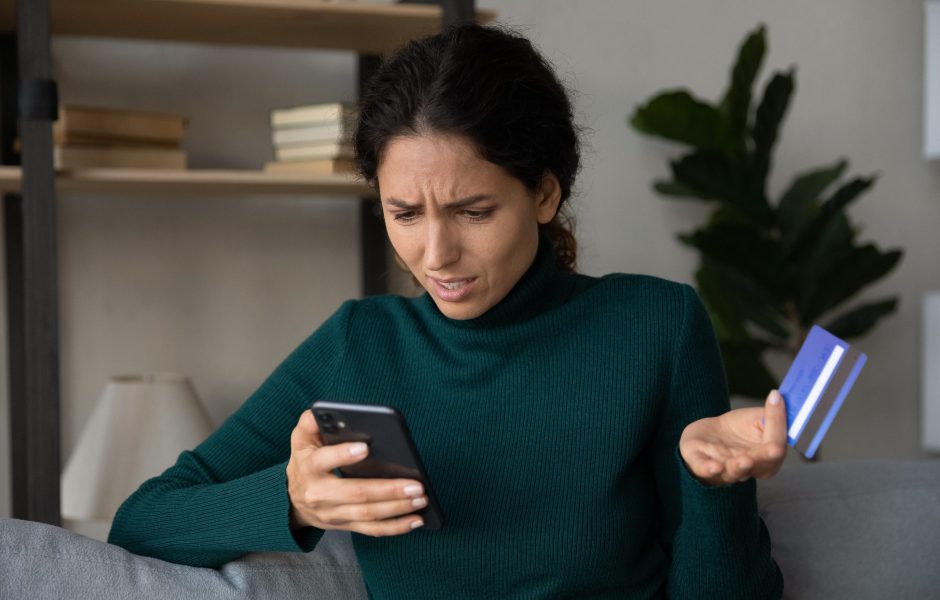
Understanding False Positives
False positives, in the context of eCommerce fraud protection, occur when transactions from legitimate customers are incorrectly identified as fraudulent. These false declines are due to the inherent complexity of distinguishing between lawful and illicit activities within a vast sea of transactions.
Essentially, false positives represent the errors made by detection systems or human monitors in their attempt to flag suspicious behavior. Striking a balance between precision and recall—the ability to accurately identify fraud without producing an excessive number of false positives—poses a perpetual challenge for organizations invested in merchant fraud protection.
Causes of False Positives
Common causes of false positives include:
Inherent Limitations of Detection Systems
Detection systems, often powered by advanced algorithms and machine learning, play a pivotal role in identifying patterns indicative of fraud. However, these systems are not infallible. False positives may occur due to the inherent limitations of algorithms, which may struggle to adapt to emerging fraud techniques or exhibit sensitivity to unusual but legitimate transaction patterns. Balancing the need for sensitivity to detect potential fraud with the requirement for specificity to minimize false positives remains a constant challenge for organizations deploying these technologies.
Human Error in Monitoring
Despite the sophistication of automated systems, human manual review involved in monitoring and reviewing flagged transactions remains crucial. However, human monitors are susceptible to errors, such as misinterpretation of data, cognitive biases, or fatigue. In some cases, a lack of expertise in understanding evolving fraud tactics can contribute to misjudgments. Addressing these human-centric challenges requires continuous training, development, and the implementation of effective oversight mechanisms to enhance accuracy in the decision-making process.
Evolving Fraud Techniques
Fraudsters continually adapt and refine their techniques, leveraging technological advancements to stay one step ahead of detection systems. As fraud tactics become more sophisticated, detection systems may struggle to keep pace, leading to an increased risk of false positives. Understanding the dynamic nature of fraud and regularly updating detection mechanisms to align with emerging threats is imperative for organizations seeking to minimize false positives while maintaining effective fraud prevention measures.
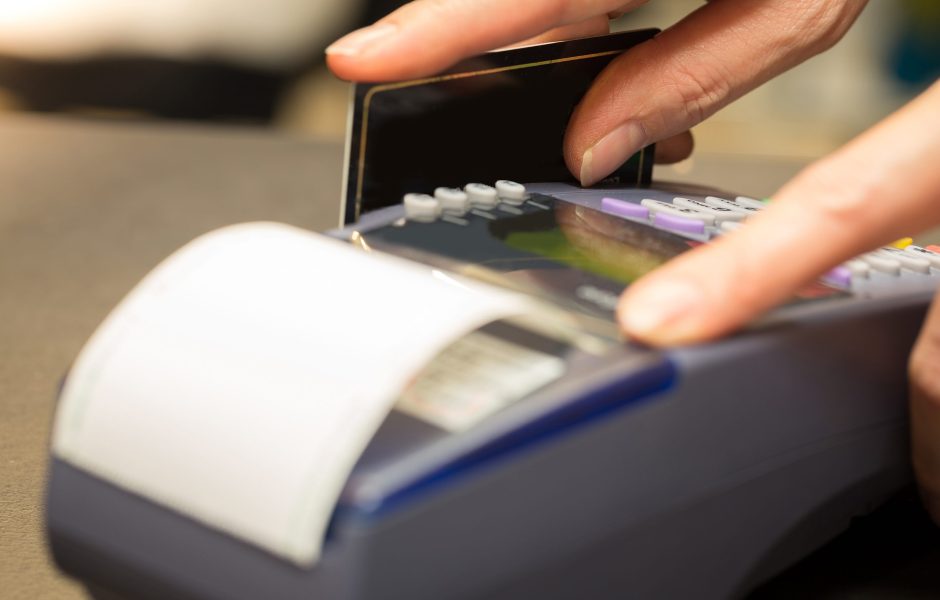
Impact on Retailers and Individuals
Understanding the multifaceted impact of false positives on both financial and reputational aspects is crucial for businesses and individuals alike. As organizations strive to fortify their fraud detection measures, they must also consider the broader consequences of false positives to strike a balance that ensures security without compromising the overall experience for customers.
Financial Consequences
The repercussions of false positives extend beyond mere inconveniences, as they can inflict significant financial burdens on both businesses and individuals. When legitimate transactions are erroneously flagged as fraudulent, it can lead to delays, declined transactions, revenue loss, and even the freezing of assets. Businesses with a high false positive rate may incur losses due to the rejection of valid customer transactions, impacting revenue streams. Additionally, the costs associated with investigating false positives and rectifying errors further contribute to the financial strain on organizations.
Reputational Risks
False positives in fraud detection can tarnish the reputation of businesses and financial institutions. Customers may perceive frequent false alarms as a sign of inefficiency or unreliability, eroding trust in the organization’s ability to safeguard their transactions. The negative perception generated by false positives can result in customer dissatisfaction, leading to a loss of loyalty and potential damage to the brand’s image. As reputation is a cornerstone of success in the business world, mitigating the impact of false positives becomes essential for maintaining customer trust.
Customer Experience
False positives directly impact the experience of customers attempting to carry out legitimate transactions. Frustration mounts when transactions are unjustifiably flagged, leading to inconvenience and potential harm to the customer-business relationship. Cumbersome processes for resolving false positives, such as lengthy verification procedures, may further exacerbate customer dissatisfaction. In an era where seamless and secure transactions are paramount, the negative impact on customer experience can have lasting consequences, influencing customer retention and acquisition efforts.
Navigating the Complex Landscape
Addressing the challenge of false positives in fraud detection requires a delicate balancing act between precision and recall. Precision involves accurately identifying fraud while minimizing false positives, ensuring that flagged transactions are indeed illicit. Recall, on the other hand, measures the system’s ability to identify all instances of fraud, avoiding false negatives where fraudulent activities go undetected. Striking the right balance is crucial; an overly conservative approach may lead to more false positives, while an overly lenient one may compromise the system’s effectiveness in catching actual instances of fraud.
Human oversight remains a critical component in mitigating false positives. Human monitors can provide the contextual understanding and nuanced insights that automated systems may lack. By leveraging human expertise, organizations can fine-tune detection mechanisms, interpret ambiguous cases, and adapt to emerging eCommerce fraud trends. However, human intervention introduces the risk of errors and biases, emphasizing the need for comprehensive training and oversight to ensure effective collaboration between automated systems and human experts.
Technological Challenges in Minimizing False Positives
Machine Learning Algorithms
Artificial intelligence and machine learning algorithms form the backbone of many modern fraud detection systems. These algorithms analyze vast datasets to identify patterns indicative of fraudulent behavior. However, they face challenges in adapting to evolving fraud techniques. As fraudsters continually refine their methods, machine learning models must undergo regular updates and fine-tuning to maintain their effectiveness. Additionally, the interpretability of these algorithms poses challenges, making it essential for organizations to strike a balance between the sophistication of models and their explainability.
Rule-Based Systems
Rule-based systems operate on predefined criteria, flagging transactions that meet specific conditions. While these systems can be effective in certain scenarios, they may struggle to adapt to the dynamic nature of fraud. Rigidity in rule-based systems can lead to high false positive rates, especially when faced with emerging or sophisticated fraud techniques that deviate from predefined rules. Organizations must continually update and augment rule sets to enhance adaptability without sacrificing accuracy.
Strategies for Minimizing False Positives
Organizations must invest in continuous improvement, leverage high-quality training data, and actively participate in collaborative initiatives to stay ahead of evolving fraud threats.
Continuous System Optimization
Continuous system optimization is a fundamental strategy for mitigating false positives in fraud detection. Organizations must regularly assess and refine their detection mechanisms to adapt to changing fraud patterns and technological advancements. This involves fine-tuning machine learning algorithms, updating rule sets, and incorporating feedback from false positive cases. By adopting an iterative approach to system optimization, businesses can enhance the accuracy of fraud detection while minimizing the impact on legitimate transactions.
Enhanced Training Data
The quality of training data significantly influences the effectiveness of fraud detection systems. Organizations should prioritize the collection of diverse and representative datasets that encompass a wide range of legitimate and fraudulent transactions. This approach ensures that machine learning models and rule-based systems are exposed to the complexity and diversity of real-world scenarios. Enhanced training data empowers detection systems to better discern patterns, reducing the likelihood of false positives and improving overall accuracy.
Collaborative Industry Efforts
Collaboration within the industry is a powerful strategy for tackling the challenges of false positives. By sharing eCommerce fraud statistics, insights, best practices, and data on emerging fraud trends, organizations can collectively strengthen their defenses. Industry collaborations facilitate the development of more robust detection algorithms, and rule sets that are informed by a broader understanding of evolving fraud techniques. Additionally, collaborative efforts can lead to the establishment of industry standards and guidelines, creating a unified approach to fraud prevention that benefits all stakeholders.
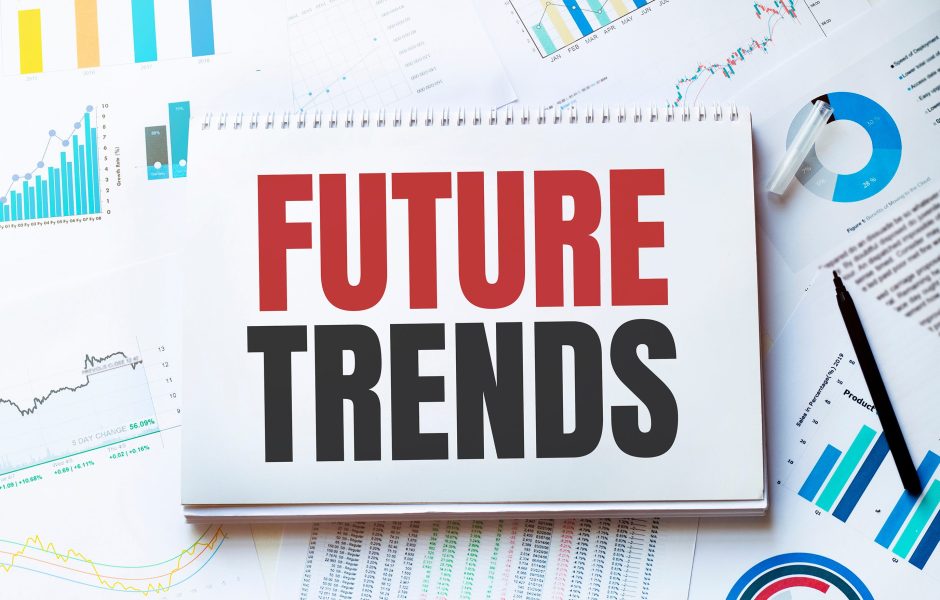
Future Trends and Innovations
As organizations look to the future, a proactive stance in adopting emerging technologies and embracing collaborative approaches will be essential to stay resilient in the face of evolving fraud challenges.
Advancements in Fraud Detection Technologies
The future landscape of fraud detection is poised for transformative advancements driven by cutting-edge technologies. Machine learning and artificial intelligence are expected to undergo continuous refinement, with increased emphasis on explainability and interpretability. Advanced anomaly detection techniques, coupled with real-time analysis, will enhance the ability of detection systems to identify subtle and evolving patterns of fraudulent behavior. The integration of behavioral biometrics, such as keystroke dynamics and mouse movement analysis, is anticipated to provide an additional layer of authentication, making it more challenging for fraudsters to bypass security measures.
Furthermore, the advent of blockchain technology holds promise for securing transactions and reducing fraud risks. The immutable and decentralized nature of blockchain can enhance the traceability and transparency of financial transactions, adding a new dimension to fraud prevention. As the digital landscape evolves, so too will the tools and technologies at the disposal of organizations committed to staying one step ahead of fraudsters.
Emerging Strategies to Tackle False Positives
The evolution of fraud detection strategies will also involve novel approaches to addressing false positives. Machine learning models will become more adaptive, capable of learning from real-time feedback and adjusting their parameters dynamically. Explainable AI will play a crucial role, providing transparency into the decision-making process of complex algorithms and instilling confidence in users and stakeholders.
Moreover, the integration of advanced analytics, including network analysis and user behavior profiling, will contribute to a more holistic understanding of transaction patterns. This multifaceted approach will enable organizations to differentiate between genuine and fraudulent activities more accurately, reducing false positives without compromising on detection efficacy.
Collaboration and information-sharing initiatives within industries will continue to evolve, fostering a collective defense against fraud. Enhanced coordination among organizations, financial institutions, and regulatory bodies will facilitate the development of standardized frameworks and the sharing of threat intelligence. This collaborative ecosystem will not only strengthen fraud prevention efforts but also provide a unified front against increasingly sophisticated fraud tactics.
Reduce False Positives With Radial
The impact of false positives on financial consequences, reputational risks, and customer experience underscores the urgency of effective fraud prevention strategies.
Radial’s cutting-edge payment solutions technologies and commitment to advancing fraud detection align seamlessly with the future trends we explored. Our comprehensive approach, incorporating machine learning, rule-based systems, and continuous optimization, positions us as a valuable partner for businesses seeking to navigate the challenges of false positives.
Radial’s dedication to enhancing the customer experience while safeguarding against fraud aligns with the industry’s evolving needs. By promoting collaboration, sharing industry insights, and staying at the forefront of technological advancements, Radial exemplifies the proactive stance necessary for effective fraud prevention.
Radial can help reduce false-positives.